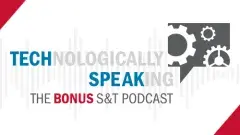
The Developmental Test and Evaluation Alarm Resolution team at the Transportation Security Laboratory is using machine learning to verify the effectiveness of newly developed explosives detection solutions. This allows new and improved detection systems to be validated and certified more quickly and efficiently, with the goal of providing safety and security at checkpoints.
Show Notes
- Transportation Security Laboratory
- Recorded date: July 26, 2023
Guests: Jason Stairs, Branch Supervisor, Developmental Testing and Evaluation Alarm Resolution, Transportation Security Laboratory (TSL)
John Devine, General Engineer, Developmental Test and Evaluation Division, TSL
[00:00:00] Jason Stairs: If you train a dog, the dog will detect exactly what you trained it to, but sort of nothing else. Well, so will the machine learn only what you trained it to detect. So, that means you just have to have so much more data to cover all of the possible scenarios of what a bomb might look like if somebody was trying to hide it on their person or in their luggage.
[00:00:20] Dave: Hi I'm Dave, editor for S&T and welcome to Tech Speak, a mini episode of the Technologically Speaking podcast. You just heard Dr. Jason Stairs, branch supervisor of S&T's Transportation Security Laboratory, or TSLs, Developmental Testing and Evaluation Alarm Resolution team. Today, we are checking in with the T S L and the work they're doing with machine learning.
[00:00:41] Jason Stairs: Machine learning is at the simplest level, just computers, machines, learning how to do a task of some sort. But a lot of times it's pattern recognition. And it's only become possible, I'd say in, in recent history. They've been talking about it just back in the sixties, if not before, maybe the early 1900s, the possibilities of machines learning. But there was never enough processor speed. You could tell it what the data is. That's called supervised learning. And from then on, if it trains properly, whenever it sees that data, it'll know what it is and can tell you what it is. So, whether that's speech or text or image recognition, I mean, machine learning I think is a big part of artificial intelligence and it's all around us at this point. Our job is to collect data on explosives. And we're one of the only places that does that. So, we have all the data and now we can take that data and try to teach machines to detect explosives, for example. And I read something recently that the calculations that that could be done in a G P U or something or on the level one of the larger machine learning models that were ever made was a neural network, and it basically had the equivalent number of neurons as a dog. So, if you could wrap your head around that. But just like we can teach dogs to detect explosives and the dogs will detect the explosives, but just exactly the ones that you trained them to detect, it's not going to smell a new explosive and know that that's an explosive. Machine learning is kind of the same way the computer will learn exactly what you teach it and sort of nothing else and, and that's the challenge that we're trying to deal with here at the T S L from a test and evaluation perspective, because we're going to have to test these machine learning models that are coming in on industry developed equipment, and now we have to, test them to make sure that they detect the explosives and not just one kind of explosives, but all of the explosives that need to be detected.
[00:02:43] Dave: We asked Jason about the future of machine learning and how it will shape new technology.
[00:02:48] Jason Stairs: Well, I think it already is. We as a species are giving these machines all of our data and then we use their applications and they get more data. And from all of that, they're learning and they're learning what we like. They're learning what we don't like. It has really speeded up things. So before when you wrote code, you had to tell the machine, if you see something that looks exactly like this, that's exactly this color or shape, then you could say that's an explosive, for example. And that's, really labor intensive and hard to do to again, get all the different variations that you need to in that engineered code. But a machine can look at that millions of times in a second and start to see patterns that we would never see as a human. So, it can really help get things done faster. But it's really going to accelerate development because it's almost, it's just so easy to do and the sky's the limit, I think. So, I mean, we'll see it everywhere. If we're talking specifically about what we do here on base at the T S L. The companies that are developing explosive detection equipment are trying to utilize machine learning in order to make their systems better. There's industry technology providers providing systems today with machine learning algorithms on them, and we are testing them and certifying them. Yes, with the hope that they would be deployed in security checkpoints. And then once they're deployed, we have to also make sure they continue to work. We provide feedback to those technology providers so that they have the best chance for success. We all want the same thing. We all want, adequate explosive detection at the airport.
[00:04:22] Dave: Here's John Devine. He's a general engineer also with TSL’s Developmental Test and Evaluation Division.
[00:04:28] John Devine: Hopefully revolutionizing the checkpoint environment by maximizing threat detection, but also maintaining a fast throughput for our flying public. The biggest challenge right now from our standpoint is the quantity and quality of data that we have. And related to that, any unforeseen biases or favoritism that we may have in our data set that we're not aware of, all that's could potentially combine for unforeseen outcomes from our trained models. And a potential solution to, you know, the data set problem would probably be synthetic data, but how you create and validate synthetic data opens up its whole new world of problems going back to the beginning. So, partnering with other branches of government, partnering with the industry. Hopefully, we can solve those problems together. Synthetic data is really data that didn't come from a physical object going through a system. It could have started that way, but whether it be through manipulation in the backend or just created from the ground up digitally, synthetic data is just data that was created or augmented on a computer as opposed to coming straight off of a system. Our specific hope for machine learning and also just products that come out of the T S L, like machine learning hasn't really changed the scope or our mission.
[00:05:49] Dave: Keeping us all safe when we travel is a vital part of the mission at DHS and the Transportation Security Laboratory’s Developmental Testing and Evaluation Alarm Resolution team is using all the tools at their disposal to make travel safer and smoother. This has been Tech Speak. Thank you for listening. You can learn more about the T S L on S&T's website and by following us on social media at DHS Scitech DHS, S C I T E C H. Bye.